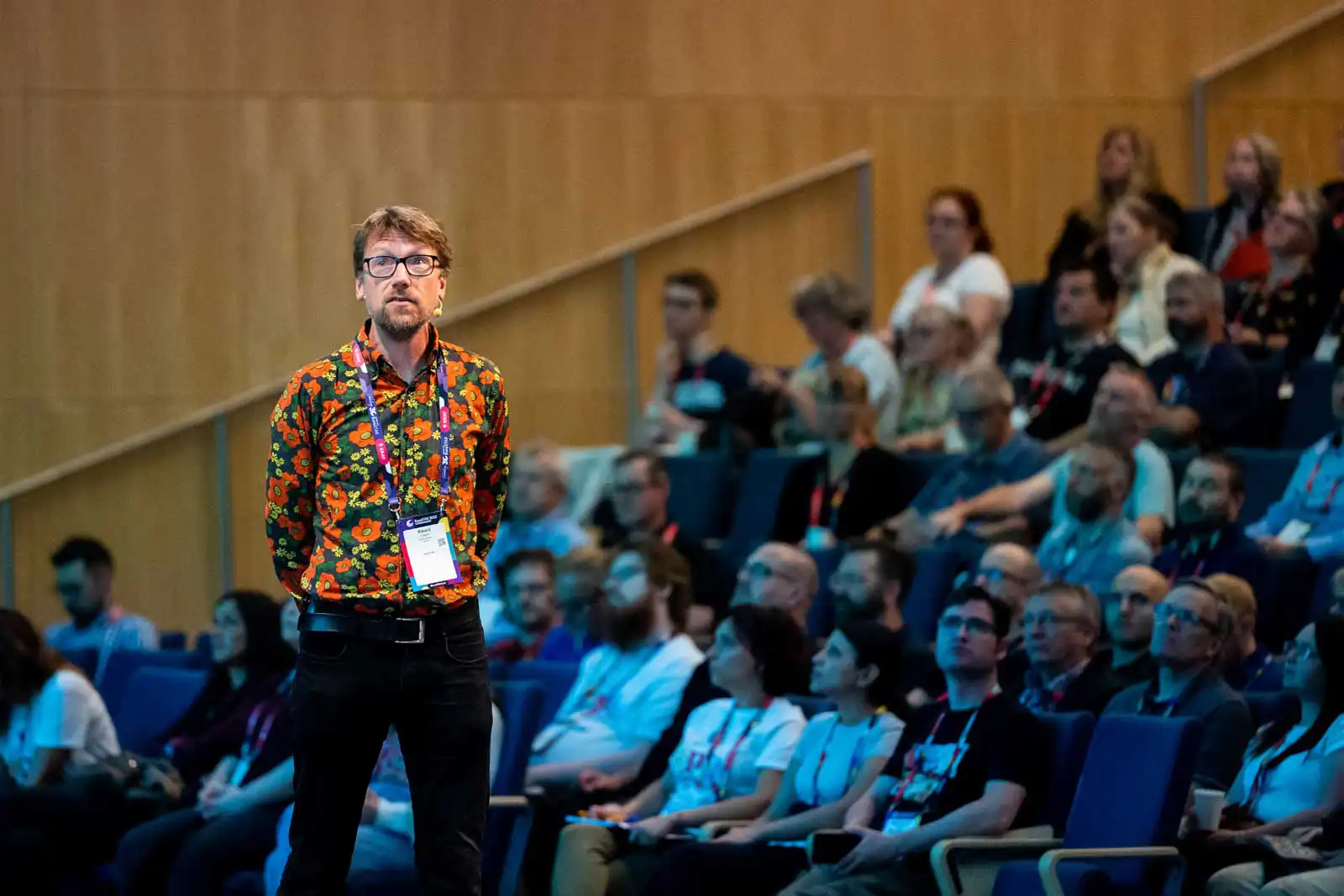
Past Events by EuroSTAR
EuroSTAR is Europe’s leader in events for software testers. Over the past 30 years we’ve hosted many different conferences including EuroSTAR, AsiaSTAR, Roadshows, OdinSTAR, UKSTAR, Test & Quality Summit and AutomationSTAR. Our events are open to all software professionals – particularly those with a passion for software testing and quality assurance – regardless of your approach to testing, your chosen school of thought, your affiliations or accreditation. Check out our events below, and join us for a professional experience you’ll never forget.
EuroSTAR Online
EuroSTAR transitioned to a fully virtual experience due to the Covid-19 pandemic in 2020 – but the community spirit was still flying high!
Over two virtual conferences in 2020 and 2021, our community grew even bigger with people tuning in from all over the world. We were joined by incredible expert speakers sharing insights, and even created a community choir!
UKSTAR
UKSTAR was a 2-day software testing conference that was held in London in 2017, 2018 and 2019.
#UKSTARConf was a roaring success – search the hashtag on Twitter for all the highlights! UKSTAR speakers included Angie Jones, Dan Billing, and Anne Marie Charrett. The programme was jam packed with inspiring learnings.
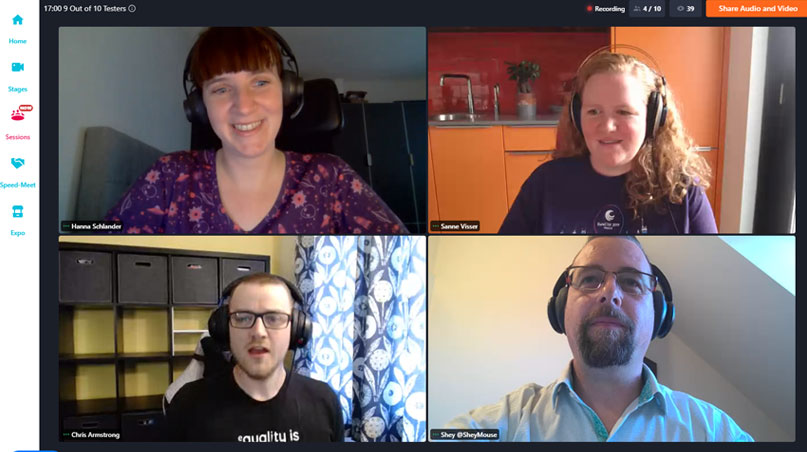
Test & Quality Summit
Test & Quality Summit was an incredible 1-day online event for test and quality team professionals. It was due to take place in-person in Dublin in April 2020, but transitioned to online in September 2020.
We welcomed leading international experts in test and quality, for an invigorating 2 days of talks on topics such as AI, Test Automation, SaFe, Valuing your Role and more.
Recent Partners
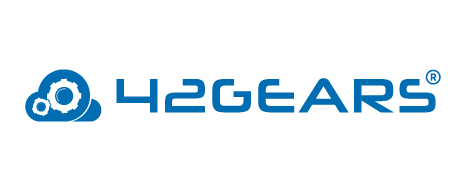
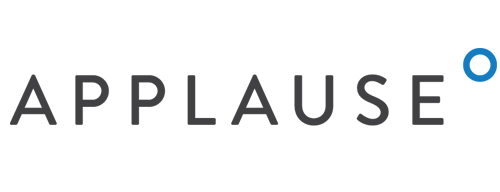
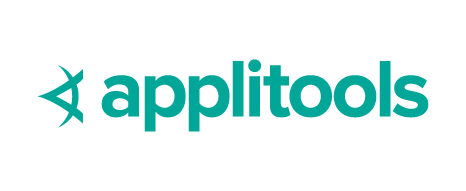
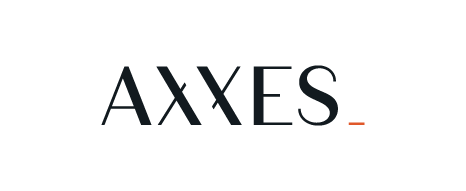
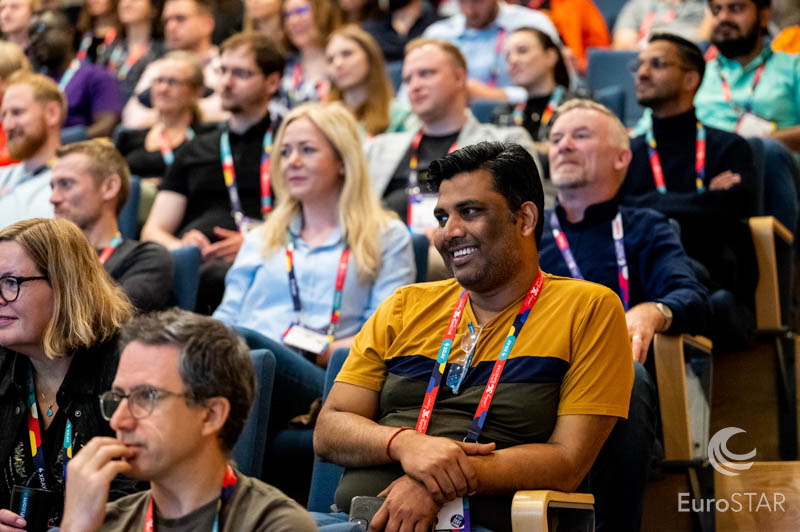
Upcoming Events
All EuroSTAR organised events are dedicated software testing conferences, where you’ll learn from so many of the best minds in software testing. Check out what’s coming up next.
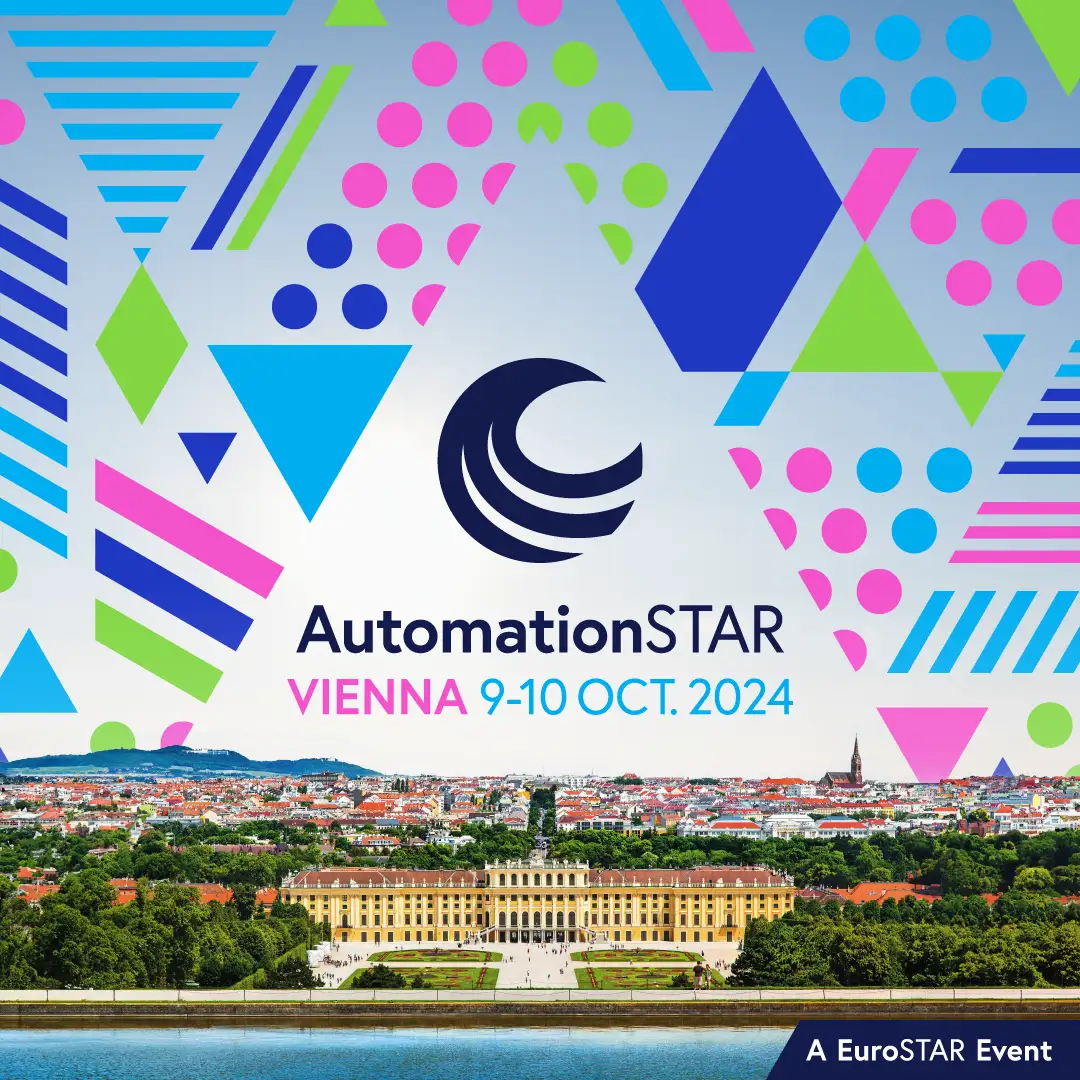
AutomationSTAR 2024, Vienna
AutomationSTAR is a 2 day conference totally focused on automation in testing. The next edition takes place in Vienna, 9-10 Oct. 2024. Enjoy training tutorials, inspiring keynotes, technical track talks, and plenty of opportunities to meet fellow automators, developers and testers.
Secure your tickets today and join 350+ attendees from over 23 countries.

Do you need approval to attend a EuroSTAR Conference?
Get in touch with us, and we’ll supply you with resources to help you get that YES.
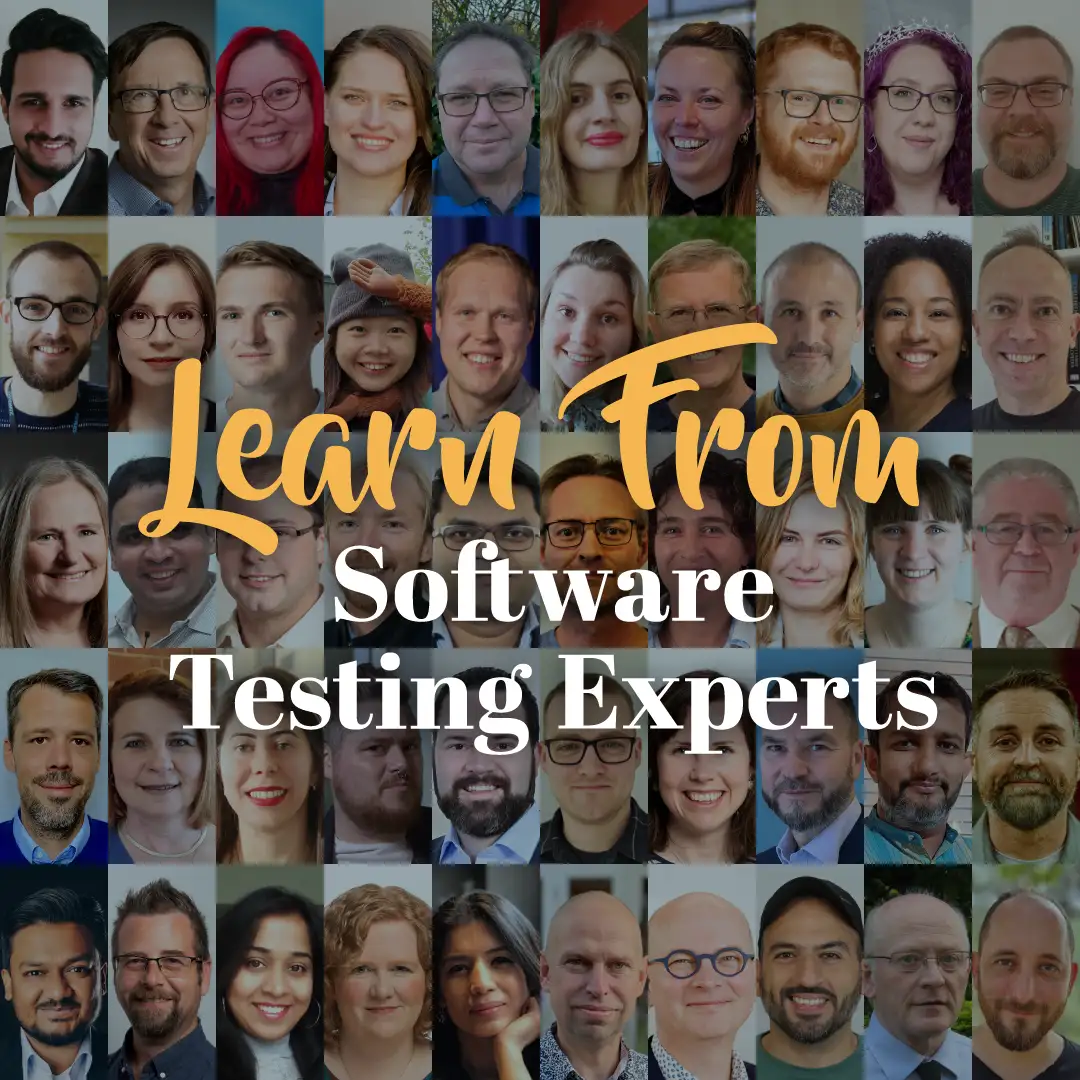
EuroSTAR 2024, Stockholm
EuroSTAR takes place in Stockholm, the beautiful capital of Sweden, 11-14 Jun. 2024. The theme is ‘What Are We doing Here?’ and Michael Bolton is the EuroSTAR 2024 Programme Chair? There will be 65+ experts speakers ready to share their knowledge.
Book your ticket now, and if you bring your whole testing team, you save almost 50% based on Super Early Bird prices and teams bundles.
Why attend EuroSTAR events
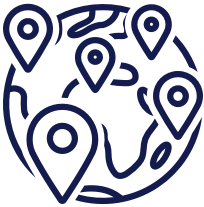
Global connections
Hear new thoughts, validate your ideas & change your perspectives with people who tackle the same problems. Meet over coffee, converse in the Huddle area, & collaborate in tutorials.
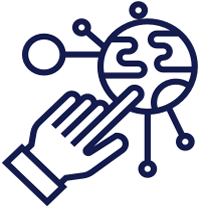
Gain knowledge
Each EuroSTAR event programme is packed with content from Europe’s testing trailblazers. No matter what area in testing you work in, you’ll learn new skills and get new ideas to apply to your projects
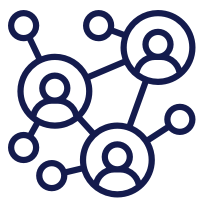
Solve testing challenges
Our testing experts are here to help! Bring your toughest questions and testing challenges to the community: get 1:1 help from speakers, collaborate with your peers in tutorials or over coffee, and solve problems together.
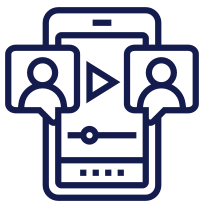
Explore new tools
A EuroSTAR organised event always draws some of the biggest companies in the world, and the EXPO is no different. Stay on top of what’s coming next in testing tools, check out the demos, and solve testing issues.
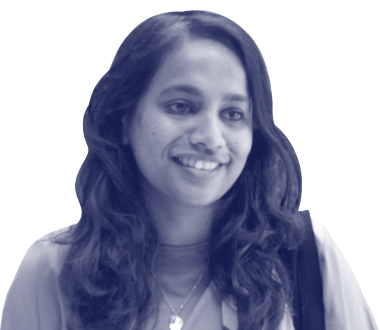
“The most important thing about EuroSTAR has been the fresh perspectives I’ve gained on different concepts of testing. I enjoyed all the talks – it’s been a great pleasure. I’m already planning my next one!”